1. Introduction:
Grok 3 was recently released, and I was particularly interested in testing its "deep search" capability. As a medical resident, I wanted to evaluate its potential as a research assistant, especially since they've made it temporarily accessible to free users.
2. Testing Methodology
A fundamental challenge with AI models is their tendency to hallucinate—generating false or non-existent information. This is particularly concerning in medicine and other fields where accuracy is crucial.
There are typically two ways to identify AI hallucinations, in my opinion:
- Having deep expertise in the subject matter (which is somewhat counterintuitive since we often use AI to learn about unfamiliar topics)
- Verifying the provided sources, which is particularly relevant for Grok's "deep search" feature
For my evaluation, I chose to focus on a specific medical topic: the use of corticosteroids in Acute Respiratory Distress Syndrome (ARDS). Specifically, I wanted to examine published Randomized Controlled Trials (RCTs) regarding their efficacy and safety.
3.Results
The model conducted its search across 35 webpages over 2 minutes and 16 seconds before providing the following results:
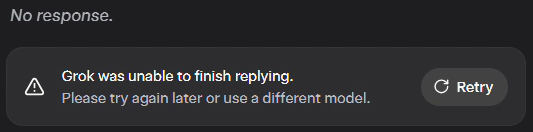
Yes it crashed!
So I tried again and it took 1min 54s for the results, they are listed below:
Overview of grok-3's response:
Key Points
- There are 14 published Randomized Controlled Trials (RCTs) on the safety and efficacy of corticosteroids in Acute Respiratory Distress Syndrome (ARDS).
- These studies include both adult and pediatric patients, with some focusing on COVID-19-related ARDS.
- Some studies also cover related conditions like septic shock, which may include ARDS patients.
Overview
This response provides a comprehensive list of all published RCTs examining the safety and efficacy of corticosteroids in ARDS, ensuring only RCTs are included as requested. The studies span various patient groups, including adults and children, and some address ARDS in the context of COVID-19. Notably, some trials also include patients with related conditions like septic shock, which may overlap with ARDS cases.
Below is the list of 14 RCTs, each with full citations for easy reference:
- Meduri GU, et al. Methylprednisolone infusion in early severe ARDS: results of a randomized controlled trial. Chest. 2007;131(4):954-963. PMID: 17426195
- Tongyoo S, et al. Hydrocortisone treatment in early sepsis-associated acute respiratory distress syndrome: results of a randomized controlled trial. Critical Care. 2016;20(1):329. PMID: 27784335
- Villar J, et al. Dexamethasone treatment for the acute respiratory distress syndrome: a multicentre, randomised controlled trial. The Lancet Respiratory Medicine. 2020;8(3):267-276. PMID: 31928907
- Annane D, et al. Effect of low doses of corticosteroids in septic shock patients with or without early acute respiratory distress syndrome. Critical Care Medicine. 2006;34(1):22-30. PMID: 16374157
- Steinberg KP, et al. Efficacy and safety of corticosteroids for persistent acute respiratory distress syndrome. The New England Journal of Medicine. 2006;354(16):1671-1684. PMID: 16625008
- Jamaati H, et al. No clinical benefit of high dose corticosteroid administration in patients with COVID-19: a preliminary report of a randomized clinical trial. European Journal of Pharmacology. 2021;897:173947. PMID: 33647378
- Tomazini BM, et al. Effect of dexamethasone on days alive and ventilator-free in patients with moderate or severe acute respiratory distress syndrome and COVID-19: The CoDEX Randomized Clinical Trial. JAMA. 2020;324(13):1307-1316. PMID: 32876697
- Drago BB, et al. Double-blind, placebo-controlled pilot randomized trial of methylprednisolone infusion in pediatric acute respiratory distress syndrome. Pediatric Critical Care Medicine. 2015;16(3):e74-81. PMID: 25647114
- Bernard GR, et al. High-dose corticosteroids in patients with the adult respiratory distress syndrome. The New England Journal of Medicine. 1987;317(25):1565-1570. PMID: 3317054
- Rezk NA, Ibrahim AM. Effects of methyl prednisolone in early ARDS. Egyptian Journal of Chest Diseases and Tuberculosis. 2013;62(3):519-524. DOI: 10.1016/j.ejcdt.2013.05.005
- Liu L, et al. The effect of stress dose glucocorticoid on patients with acute respiratory distress syndrome combined with critical illness-related corticosteroid insufficiency. Chinese Journal of Internal Medicine. 2012;51(8):599-603. PMID: 23158856
- Confalonieri M, et al. Hydrocortisone infusion for severe community-acquired pneumonia: a preliminary randomized study. American Journal of Respiratory and Critical Care Medicine. 2005;171(3):242-248. PMID: 15516528
- Meduri GU, et al. Effect of prolonged methylprednisolone therapy in unresolving acute respiratory distress syndrome: a randomized controlled trial. JAMA. 1998;280(2):159-165. PMID: 9669789
- Seam N, et al. Effects of methylprednisolone infusion on markers of inflammation, coagulation, and fibrinolysis in patients with severe sepsis or acute respiratory distress syndrome. Annals of Intensive Care. 2012;2(1):49. PMID: 23210475
I wanted to test it more but unfortunately, I reached my limit as a free user and I have to pay 30$/month to use the "deep search" feature in what they call supergrok. In my opinion it's quite expensive but it's less expensive than the 200$/month for the pro version of openai's chatGPT.
4.Evaluating Grok's Search Accuracy:
Search Performance
The model completed its search in 1 minute and 54 seconds, yielding good but mixed results. Let's break down this performance into its strengths and limitations:
Strengths
The model demonstrated impressive accuracy in identifying core bibliographic elements:
- Successfully captured 13 out of 14 study titles with perfect accuracy
- Nailed all 14 first-author names (100% accuracy)
- Correctly identified publication details (journal and date) for 13 out of 14 studies
Limitations
However, some notable inconsistencies emerged:
- The final study entry showed partial inaccuracies:
- Title was partially misrepresented
- Journal of publication was incorrectly cited
- Though interestingly, both first author name and publication date remained accurate
- PMIDs showed significant discrepancies:
- Only 4 papers had correct PMIDs
- The remaining identifiers were inaccurate
This mixed performance highlights both the promising potential and current limitations of AI-powered research tools. While the high accuracy in capturing basic bibliographic information is encouraging, the inconsistencies in unique identifiers like PMIDs suggest there's still room for improvement in data verification and cross-referencing capabilities.
5.Comparing AI Performance with Human Systematic Reviews:
Benchmarking Against Published Research
I've found something truly remarkable in comparing Grok 3's performance with traditional human-conducted systematic reviews. Let me break it down:
Key Reference Points
The comparison was made against two recent meta-analyses:
- Chang et al. (2022)[1]: A comprehensive systematic review and meta-analysis
- Li et al. (2024)[2]: A more recent meta-analysis
Remarkable Alignment
What's particularly fascinating is the perfect alignment between Grok 3's results and the Chang et al. study. All 14 studies identified by the human researchers were also captured by the AI model. This isn't just coincidence - it reflects the model's ability to access and process open-access content effectively.
Here you can see the paper in question being included in the research:
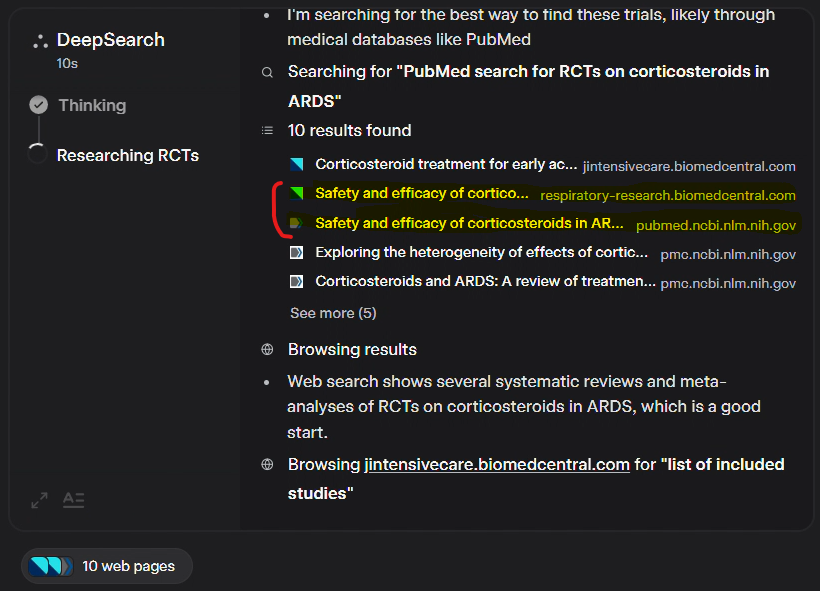
and it was explicitly stated in the final result:
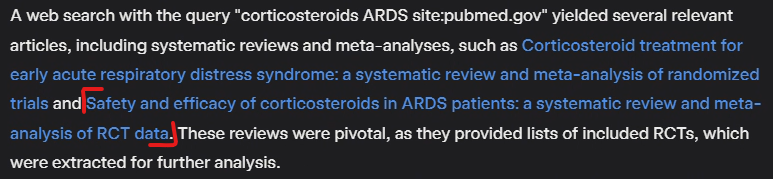
The alignment with the Li et al. (2024)[2] meta-analysis further reinforces our findings - all 9 RCTs from their study were accurately captured in Grok's results. Again, the open-access nature of this paper likely contributed to this performance.
Understanding the Context
This alignment makes perfect sense when we consider that Chang's and Li's papers are openly accessible. It's a brilliant example of how AI can leverage existing high-quality systematic reviews to rapidly synthesize information. The model's ability to identify and extract this information demonstrates both its potential and its current limitations - it's excellent at finding and processing accessible, well-structured academic content.
Note that Grok-3 didn't stop there, it searched for RCTs published after the Chang et al. paper. as illustrated below:

I wanted to highlight the rapid evolution of AI models and their capacity to understand, reason, and search for specific information. Believe me, I tried the same task with other models, and they were unable to give such a detailed response—they would tell me that they could not provide an exhaustive list and that I had to use PubMed for a manual search.
6.Implications for Research
This comparison opens up exciting possibilities for how AI could transform systematic review processes and research in general:
- Rapid initial screening of literature
- Validation of reference lists
- Cross-checking of study inclusion criteria
- Time-saving in the preliminary stages of review work
The technology isn't replacing the need for human expertise but rather amplifying our ability to conduct thorough, comprehensive reviews more efficiently.
7.Looking to the Future: Beyond Traditional Research Methods
Here's where things get really exciting! While some might ask, "Why use AI when we can directly consult systematic reviews?", this perspective misses the revolutionary potential unfolding before us. Let me explain why this test reveals something truly remarkable about the evolution of AI in medical research:
Current Breakthroughs
What sets this performance apart is the model's ability to:
- Understand complex medical queries with remarkable precision
- Execute targeted searches across multiple studies
- Synthesize information from various sources
- Provide detailed, specific responses where other models simply defer to manual searches
Imagining the Future of Medical Research
The possibilities are absolutely thrilling! Consider these potential developments:
- A PubMed integration with advanced AI search capabilities
- Specialized models fine-tuned for medical research analysis
- AI-powered statistical analysis tools for instant meta-analysis
- Real-time research assistants that can process thousands of papers simultaneously
For medical professionals and researchers, this isn't just about saving time - it's about transforming how we interact with medical literature. Imagine having a research assistant that could instantly synthesize decades of clinical trials, while maintaining the crucial element of human oversight and expertise.
8.The Bigger Picture
While we must remain vigilant about AI hallucinations and maintain rigorous verification processes, these results hint at an approaching revolution in medical research methodology. We're witnessing the early stages of tools that could dramatically accelerate the pace of medical discovery while making research more accessible and efficient than ever before.
What excites me most is not just what these tools can do today, but how they're laying the groundwork for even more sophisticated research assistance tomorrow. We're not looking to replace traditional research methods - we're enhancing them in ways that could fundamentally transform how we advance medical knowledge.
If you want me to create more content about this topic like comparing this model with other models, more detailed analysis, and other use cases in our field, let me know in the comments below and don't forget to subscribe so you won't miss any upcoming content.
References:
- Safety and efficacy of corticosteroids in ARDS patients: a systematic review and meta-analysis of RCT data.
- Efficacy of corticosteroids in patients with acute respiratory distress syndrome: a meta-analysis.
Keywords:
Grok 3, Deep search capability, Medical research, AI in healthcare, AI research assistant, Corticosteroids in ARDS, Acute Respiratory Distress Syndrome, ARDS randomized controlled trials, RCT evaluation, Systematic review automation, AI hallucinations, Bibliographic search automation, Evidence-based medicine, Clinical trial analysis, PubMed.
The information provided in this article is for educational and informational purposes only and is not intended to replace professional medical advice, diagnosis, or treatment. Always consult with a qualified healthcare provider before making any decisions related to your health, particularly if you are experiencing any symptoms or have concerns about seizure management. The author and publisher assume no responsibility or liability for any errors or omissions in the content or for any outcomes resulting from the use of this information.
Comments ()